In this article: Discover how successful engineering leaders are redefining their roles in the age of AI, with actionable strategies to help your team thrive amid rapid technological change.
I just witnessed a colleague transform from AI skeptic to advocate in 48 hours.
The turning point? Not a fancy demo or technical breakthrough.
It was seeing his team solve a problem in minutes that would have taken days before.
The Leadership Crossroads
Engineering leadership is at a crossroads. The AI revolution isn’t just changing how we code—it’s fundamentally reshaping what it means to lead technical teams. This shift demands a strategic recalibration of how we develop talent, manage workflows, and deliver value.
I’ve observed three distinct approaches emerging among engineering leaders:
- The first group treats AI as just another tool—useful but peripheral. They’re missing the forest for the trees.
- The second group goes all-in, replacing processes wholesale with AI solutions. They’re learning painful lessons about the limitations of current technology.
- The third group—the ones thriving—are taking a measured, intentional approach. They’re systematically identifying high-leverage opportunities while maintaining human oversight where it matters most.
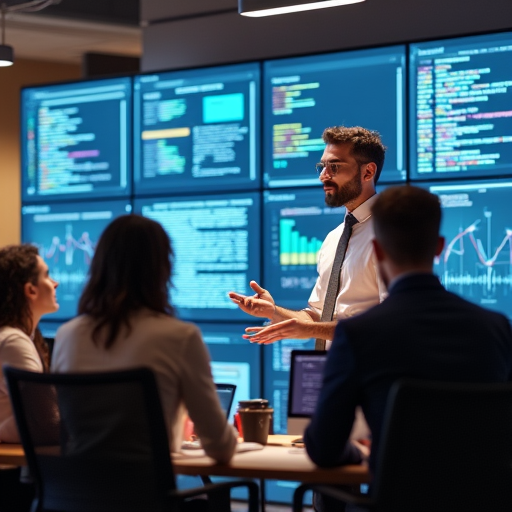
Winning Strategies for AI-Era Leaders
What separates successful engineering leaders in this AI-driven era?
Strategic AI Readiness
Rather than uniform adoption, they assess team-by-team capabilities and create tailored implementation roadmaps with clear milestones.
Real-world example: At a mid-size fintech company, the engineering director created a capability matrix for each team, identifying specific AI use cases that aligned with their current skills and domain knowledge. This targeted approach resulted in 3x higher adoption rates compared to their previous one-size-fits-all initiatives.
Redefined Productivity Metrics
Traditional lines-of-code measurements become meaningless when AI can generate thousands in seconds. Forward-thinking leaders are developing new frameworks that measure impact, not output.
Governance Before Adoption
As McKinsey research shows, clear guidelines around data security, intellectual property, and quality standards are non-negotiable prerequisites.
Embedded AI Champions
These experts bridge the gap between theoretical capabilities and practical applications in your specific domain, a strategy recommended by the Harvard Business Review.
Balanced Implementation Approach
While showcasing immediate value, successful leaders simultaneously invest in foundational skills that will compound over time, as outlined in MIT Sloan’s research on AI transformation.
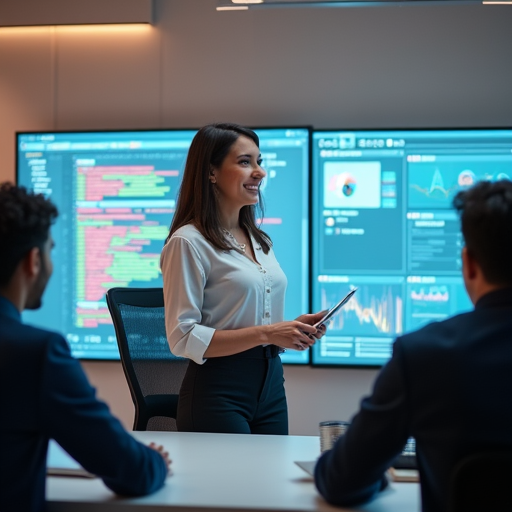
The Evolution of Leadership Itself
Perhaps most importantly, successful leaders recognize that leadership itself must evolve. The most valuable engineering leader is no longer the technical virtuoso, but the strategic orchestrator who can align AI capabilities with business objectives.
Key Takeaways
- AI adoption requires a tailored, team-specific approach
- New metrics must focus on impact rather than traditional output
- Governance frameworks should precede widespread implementation
- Embedded champions accelerate practical adoption
- Leadership skills must evolve to emphasize strategic orchestration
The question isn’t whether your engineering organization will be transformed by AI—it’s whether you’ll shape that transformation or be shaped by it.
How are you preparing your engineering teams to thrive in this new reality? Have you identified your AI champions yet? What metrics are you using to measure success beyond traditional productivity indicators?